Low Energy Sensor Platform – Leveraging Machine Learning
Abstract: Applications of embedded AI, specifically ML (Machine Learning) and object classification has
been growing exponentially as decision logic moves to the edge. Proven advantages of low energy
consumption, low costs, and independent target classification drive this implementation and is transforming
smart city management, agriculture, medical, health care, bridge and building structure health management
(SHM), and smart logistics just to name a few game-changing applications.
Several specific ML applications will be discussed including a vision ML application applied to logistics, a
remote visual cargo classification smart sensor (currently in production). Challenges of a small vs. large CNN
model when improving object identification and accuracy will also be discussed and why ML at the network
edge is a game-changer. Finally, we will compare and contrast the AI categories of large language models
and the energy-constrained tiny ML sensor models.
Bio: The speaker, Joe Jesson, co-founded & was CTO of a General Electric business, Asset Intelligence, a GE business that designed
and sold remote IoT sensors for the logistics and energy sectors. Machine learning and LPWAN sensor communication became an
integral part of the remote monitoring and management of mobile and remote assets. An ongoing research goal is to reduce the smart
energy costs where 100% of the power is generated by ambient energy harvesting. Joe is currently CEO of RF Sigint Group and has
over 25+ years of engineering and management experience with Motorola APD, Oak Technology, BP, and General Electric. Master's
degree from DePaul University & working on a Ph.D.defense. Contact: [email protected]
Note: this is a TCF Event – No need to register, but you need to purchase a TCF all-day admission ticket at https://tcf-nj.org/
Room: ED115, Bldg: Education, The College of New Jersey, Metzger Dr, Ewing, New Jersey, United States, 08618

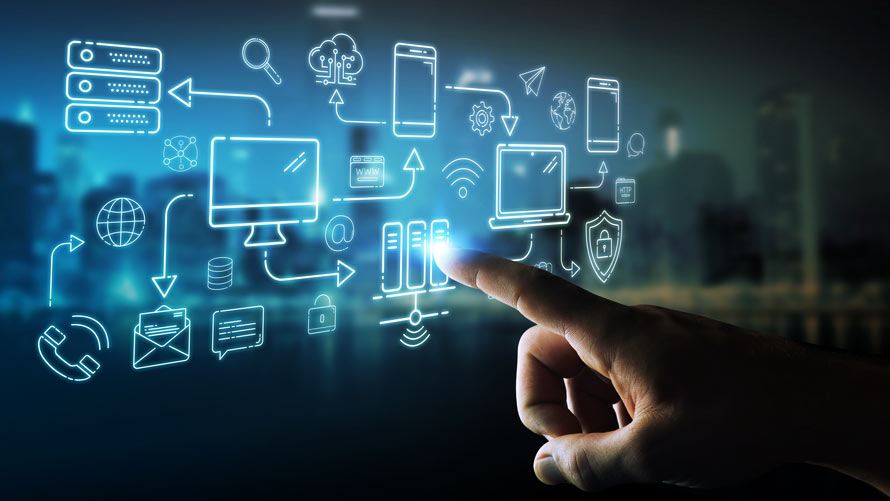