The centralization of data and intelligence poses several risks, including potential privacy breaches, single points of failure, and stifling innovation. Decentralization, on the other hand, remains challenging as edge devices often have to work with limited computing capabilities and battery energy budgets. In this talk, I will present a line of research conducted at the Faculty of Computer and Information Science, University of Ljubljana, Slovenia, where we aim to reduce the computational and energy burden of deep learning, thus enabling AI on the edge. The basis of our approach is dynamically approximable computing, which allows us to adapt the amount of computation to the actual situation in which deep learning occurs. The talk will demonstrate this philosophy applied to different domains, from physical activity recognition on smartphones to weed identification on a camera-equipped unmanned aerial vehicle (UAV).
Speaker(s): Veljko Pejovic, PhD
Room: Conference Room, University Ss Cyril and Methodius, Faculty of Electrical Engineering and Information Technologies, Rugjer Boskovikj 18, Skopje, Macedonia, Macedonia

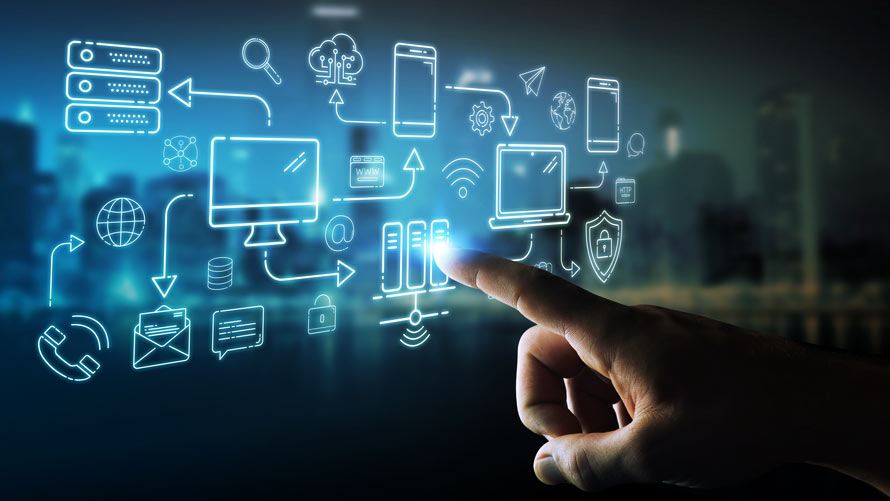